Malware Analysis Using Machine Learning: Revolutionizing Cybersecurity
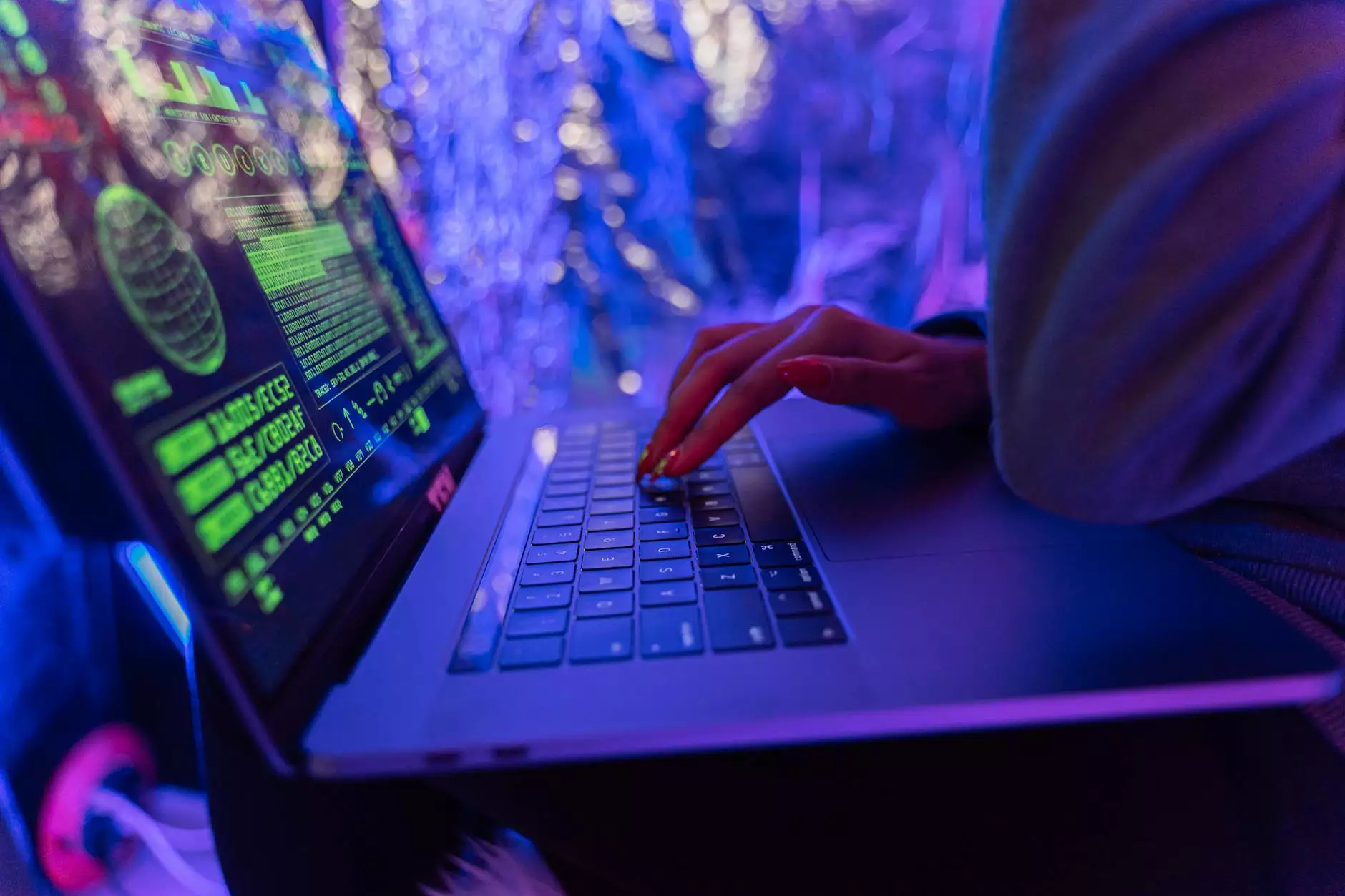
Understanding Malware and Its Implications
Malware, short for malicious software, refers to any software intentionally designed to harm, exploit, or otherwise compromise the functionality of computers, networks, or user data. The consequences of malware infections can be devastating for businesses, leading to data breaches, financial loss, and reputational damage. Thus, effective malware analysis is crucial for identifying and neutralizing threats before they can inflict harm.
The Rise of Machine Learning in Cybersecurity
With the evolving landscape of cyber threats, traditional methods of malware detection are proving insufficient. Machine learning (ML) has emerged as a pivotal tool in cybersecurity. By leveraging algorithms and statistical models, machine learning can analyze vast amounts of data to recognize patterns that are often imperceptible to human analysts.
What is Malware Analysis Using Machine Learning?
Malware analysis using machine learning combines the principles of software analysis and ML techniques to improve malware detection rates. This approach involves training machine learning models on large datasets of benign and malicious software. These models learn to distinguish between normal and suspicious behavior, allowing for real-time threat detection and response.
Benefits of Machine Learning in Malware Analysis
- Increased Detection Rates: Machine learning algorithms can identify new strains of malware more effectively than traditional signature-based methods.
- Automation: With automation, organizations can reduce the workload on cybersecurity professionals, allowing them to focus on more complex tasks.
- Adaptive Learning: Machine learning models continually evolve, improving their detection capabilities over time as they are exposed to new threats.
- Real-time Analysis: Machine learning provides rapid analyses that can identify and respond to threats as they occur.
The Role of Machine Learning Algorithms in Malware Analysis
Various machine learning algorithms play a crucial role in malware analysis. Below are some of the most commonly used:
1. Decision Trees
Decision trees are simple, yet powerful algorithms that classify malware by evaluating a series of decision nodes. They provide clear paths for decision-making, making it easy to understand why a particular software was flagged as malicious.
2. Support Vector Machines (SVM)
SVMs are effective for high-dimensional data, and they work well for malware detection by finding hyperplanes that separate data points belonging to different classes, such as benign and malicious software.
3. Neural Networks
Neural networks, particularly deep learning models, are capable of learning complex patterns in data. They have shown promising results in recognizing malware through feature extraction processes that analyze binaries and network traffic.
4. Ensemble Learning
Ensemble methods combine multiple models to improve accuracy. By aggregating the predictions from various algorithms, ensemble learning can significantly reduce false positive rates while enhancing detection capabilities.
Practical Applications of Malware Analysis Using Machine Learning
Businesses can apply malware analysis using machine learning in various ways to enhance their cybersecurity strategies:
1. Real-time Threat Detection
By implementing machine learning models, organizations can monitor network traffic in real time, identifying malicious patterns and enabling quick responses to potential threats.
2. Automated Incident Response
Machine learning can automate the response to detected threats, allowing for immediate isolation of affected systems, thus minimizing the impact of malware attacks.
3. Enhanced Forensics and Reporting
Machine learning can aid in forensic investigations by providing detailed reports on attack vectors, compromised data, and the behavior of malware, which can be crucial for future prevention strategies.
Challenges in Implementing Machine Learning for Malware Analysis
While the benefits of machine learning in malware analysis are substantial, several challenges persist:
- Data Quality: The efficacy of machine learning models relies heavily on the quality of the data used for training. Incomplete or biased datasets can lead to ineffective detection capabilities.
- Adversarial Attacks: Cybercriminals are continually evolving their tactics, creating polymorphic malware that can evade traditional detection methods, including machine learning algorithms.
- Resource Intensity: Training machine learning models can be resource-intensive, requiring significant computational power and expertise.
- Interpretability: Understanding how machine learning models arrive at their conclusions can be challenging, making it difficult for cybersecurity teams to trust and act on model predictions.
Future Trends in Malware Analysis Using Machine Learning
The landscape of cybersecurity is constantly changing, and future trends in malware analysis using machine learning are shaping up to create a safer digital environment:
1. Use of Artificial Intelligence
Advancements in artificial intelligence (AI) and machine learning will continue to enhance threat intelligence platforms, allowing for more sophisticated detection mechanisms that can predict and visualize attack trajectories.
2. Cloud-based Security Solutions
As businesses increasingly move to the cloud, cloud-based security solutions that employ machine learning for malware detection will become more prevalent, providing flexible, scalable protection against cyber threats.
3. Improved Collaboration and Information Sharing
In order to have effective malware analysis using machine learning, it is essential for organizations to collaborate and share threat intelligence, augmenting the datasets used for training machine learning models.
4. Privacy-Preserving Machine Learning
As data privacy becomes more critical, techniques such as federated learning will allow for collaborative model training while preserving the privacy of sensitive information, providing a secure way to enhance model performance.
Conclusion: The Imperative of Malware Analysis Using Machine Learning
In today’s digital age, the importance of malware analysis using machine learning in business cannot be overstated. As threats evolve, organizations must adopt advanced analytical techniques to protect their resources effectively. By leveraging the power of machine learning, companies can significantly enhance their cybersecurity posture, minimize risks, and safeguard sensitive data from malicious entities.
For businesses looking to stay ahead of the curve in cybersecurity, implementing these advanced techniques in malware analysis will not only protect against current threats but also prepare them for the challenges of the future.
Selecting the Right Partner for Malware Analysis
Choosing a proficient partner like Spambrella for IT services and computer repair as well as security systems can pave the way for comprehensive malware analysis using machine learning. Spambrella boasts a team of experienced professionals who are equipped to provide tailored solutions that align with your business’s unique needs.
Invest in the future of your business by embracing machine learning in malware analysis. The right tools and knowledge will empower your organization to navigate the complex landscape of cybersecurity with confidence.